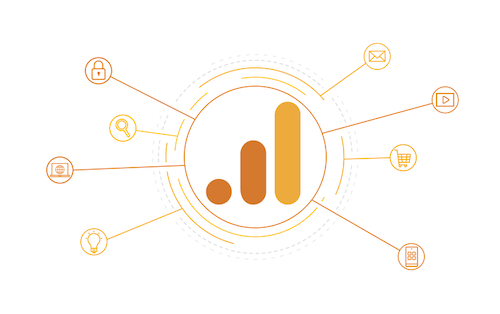
While there are a lot of discussions about good analytics, how do we recognize bad analytics? Bad analytics is more than not having good insights. Bad analytics is present when our work doesn’t support evidence-based and data-driven decision-making (3DM) for improved business performance. So, unless one can identify bad analytics within a company, it cannot be prevented. Here are 11 key characteristics of bad analytics..
1. Fundamentally, analytics is defined as using data to answer business questions so as to derive insights. If the insights derived do not answer the questions, the insights have NOT clearly addressed the business objectives, i.e., the problem or the opportunity.
2. Bad analytics will have insights prone to bias. Bias is a tendency, inclination, or prejudice towards or against something or someone. Biases are often based on stereotypes and result in poor decisions as the insights get skewed in a certain direction. Against this backdrop, the insights derived from analytics can be biased for the following key reasons
- Confirmation Bias: A confirmation bias involves insights that confirm previously existing beliefs or findings or hypotheses. Confirmation bias is presenting insights, or the question itself, in a manner one wants to see, rather than objectively deriving the insights. This type of bias usually happens when the analytics team is not very close to the business problem or the opportunity. Instead of challenging pre-existing insights or beliefs, the team prefers to play it safe by accepting the status quo.
- Availability Bias: The two main obstacles in most analytics projects are a strong hypothesis statement and good quality data. A strong hypothesis is defined by an experienced individual who brings strong business and data knowledge. Getting the time of such an individual is always a challenge in most analytics projects as their expertise is sought by many different initiatives in the company. The second challenge is a lack of quality data. Research presented in the Harvard Business Review (HBR) says that just 3 percent of the data in a business enterprise meets Data Quality standards (Nagle et al., 2017). Given the above challenges, analytics projects are forced to work with whatever is at their disposal. Availability bias refers to the way in which the derived insights are carried out using only hypotheses and data that are easily and readily available.
- Selection Bias: As getting a good amount of population data to run analytics models is difficult, analytics projects often rely on sample data. But if the data sample selected does not reflect the actual population data set, we have selection bias. This means the sample data selected is low in count, does not represent the characteristics of the population data set, and is not randomly selected. The result of selection bias is a distortion of the insights derived from statistical analysis due to a poor sampling of data, thereby resulting in bad analytics.
- Anchoring Bias: Often, people tend to rely heavily on the first piece of insight that comes across and set the standard for subsequent insights that will be derived. Anchoring bias is fixating on the initial set of insights derived and failing to adapt for subsequent insights. Given that businesses constantly evolve and collect a lot of data these days, anchoring bias is an important issue if insights are not constantly updated or refined using the most recent data.
- Framing Bias: In psychology and decision theory, loss aversion is people’s tendency to prefer avoiding losses to acquiring equivalent gains; it is better not to lose $5 than to find $5. In other words, humans are likely to avoid risks when a positive frame is presented but seek risks when a negative frame is presented. Framing bias is the way a problem is formulated or defined to avoid risk-taking, and this type of bias typically happens when there is a homogenous set of stakeholders who work and think alike.
- Sunk-Cost Bias: In business, a sunk cost is the cost that has already been incurred and cannot be recovered. Sunk-cost bias is the tendency to “honor” those insights because of resources already spent, especially time, effort, and money.
- Authority Bias: Authority bias is accepting the highest-paid person’s opinion (HiPPO) as insights. The highest-paid person usually has the most power and the highest designation in the room. Once his or her opinion is presented, dissent is shut out, thereby preventing a thorough analysis of the problem and the solution.
Although bias is hard to avoid, here are three key strategies to prevent it:
- Given that questions drive the analytics process, pose problems in a neutral manner by reframing the problem in different time frames with different stakeholders in different contexts.
- Perform exploratory data analysis techniques and summarize the key characteristics of data so as to select the best data to feed the analytics model. In addition, run different analytics algorithms on the data by reframing the question and identify the best analytics algorithm to derive insights.
- Review the decision-making process and the assumptions made to get a holistic picture of how the insights are consumed. Understanding the decision-making process will help understand how decisions are made based on the values, preferences, and beliefs of the decision-makers.
3. Bad analytics is comprised of insights derived from a poor quality of data. As mentioned earlier, often, it is very difficult for the business to get good quality data for analytics. The “Garbage-in, Garbage-out” concept is very much applicable for analytics. If the quality of data used in analytics is poor, the insights will also be of bad quality.
4. If non-normalized data is used for business insights, then it generates bad analytics. Normalized data ensures the data will be within a certain range as business processes, which typically capture discrete and continuous events shown to follow normal data distribution. In short, business processes inherently adhere to consistency and follow the normalized bell curve. If there are any exceptions, businesses will fix these variations as they despise inconsistency.
5. Bad analytics does not address outliers. Outliers are observations that are not following the same pattern as the other data sets. Outliers are not necessarily a bad thing all the time in business, as fraud analytics is heavily dependent on outliers. If the outliers are not identified and explained, this results in bad analytics. And if the outliers are eliminated, one should try to understand why they appeared and whether it is likely similar values will continue to appear.
6. Bad analytics exhibits overfitting and underfitting. Underfitting means the analytics model gives an overly simplistic picture of reality, and overfitting is when the model is overcomplicated. Bad analytics occurs when the analytics model is not validated with cross-validation (with training and test data) and multiple algorithms.
7. Bad analytics presents a potentially confounding variable in the analytics model, explaining the insight. A confounding variable is an “extra” variable that was not accounted for in analytics. In other words, confounding variables are extra independent variables that are having a hidden effect on the dependent variables.
8. Mixing correlation and causation is bad analytics. Correlation describes the relationship between two variables, while causation speaks to the idea that one event is the result of the occurrence of the other event. It is common to assume causation when there is simply a correlation in the data, and this typically happens when individuals working on the data are influenced by past experience and personal biases.
9. If the insights derived are obvious, then we have bad analytics. In other words, analytics is solving a problem based on testable hypotheses using good quality data. Overall analytics is an expensive process. Spending time in reconfirming an obvious insight or a natural phenomenon is a waste of business resources. For example, if a crude oil pipeline company after analyzing thousands of crude oil nomination tickets determines that crude oil viscosity affects the crude oil transportation time, it is not a new insight. In other words, it results in bad analytics as it is “solving” a physics problem and not a data problem.
10. The insights are not monetizable. Insights derived should have a positive outcome on the business performance. If the insights derived are not increasing revenue, reducing cost, and minimizing the risk for the business, we have bad analytics.
11. If the insights coming out of bad analytics cannot be implemented with the available resources, then we have bad analytics. Securing new or additional resources for business is always a challenge. If the insights derived cannot be implemented with the available resources, the insights derived are not very useful.
Overall, bad analytics are present when our work doesn’t support evidence-based and data-driven decision-making (3DM) for improved business performance. Analytics should be designed for a purpose. The best way to avoid bad analytics is to work on real problems for actual customers or stakeholders. In other words, tie our stakeholder’s insight needs to business goals, questions, and quality data.
Matt Joyce also contributed to this article.
References
- Nagle, Tadhg; Redman, Thomas, and David Sammon, “Only 3% of Companies’ Data Meets Basic Quality Standards”, https://hbr.org/2017/09/only-3-of-companies-data-meets-basic-quality-standards, Sep 2017
Not a member-scholar yet? Join our financial community here!
Identify your path to CFO success by taking our CFO Readiness Assessmentᵀᴹ.
For the most up to date and relevant accounting, finance, treasury and leadership headlines all in one place subscribe to The Balanced Digest.